
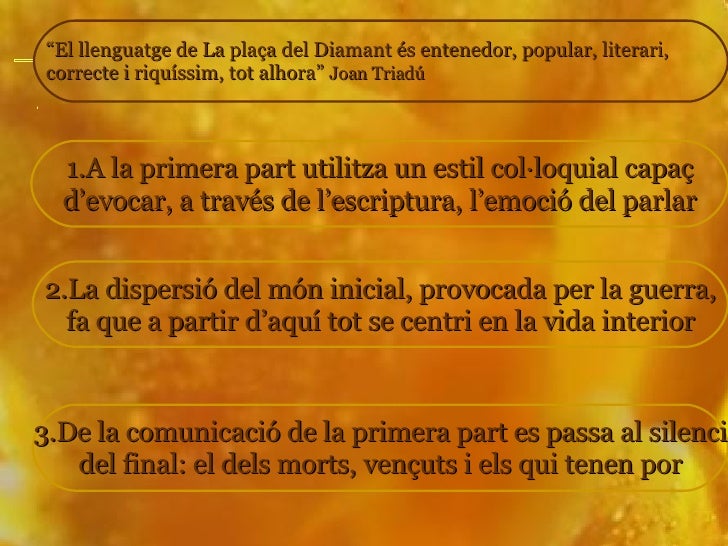
BBSeq models the dispersion on the mean, with the mean absolute deviation of dispersion estimates used to reduce the influence of outliers. Our DESeq method detects and corrects dispersion estimates that are too low through modeling of the dependence of the dispersion on the average expression strength over all samples. edgeR, moderates the dispersion estimate for each gene toward a common estimate across all genes, or toward a local estimate from genes with similar expression strength, using a weighted conditional likelihood. Many methods for differential expression analysis of RNA-seq data perform such information sharing across genes for variance (or, equivalently, dispersion) estimation.
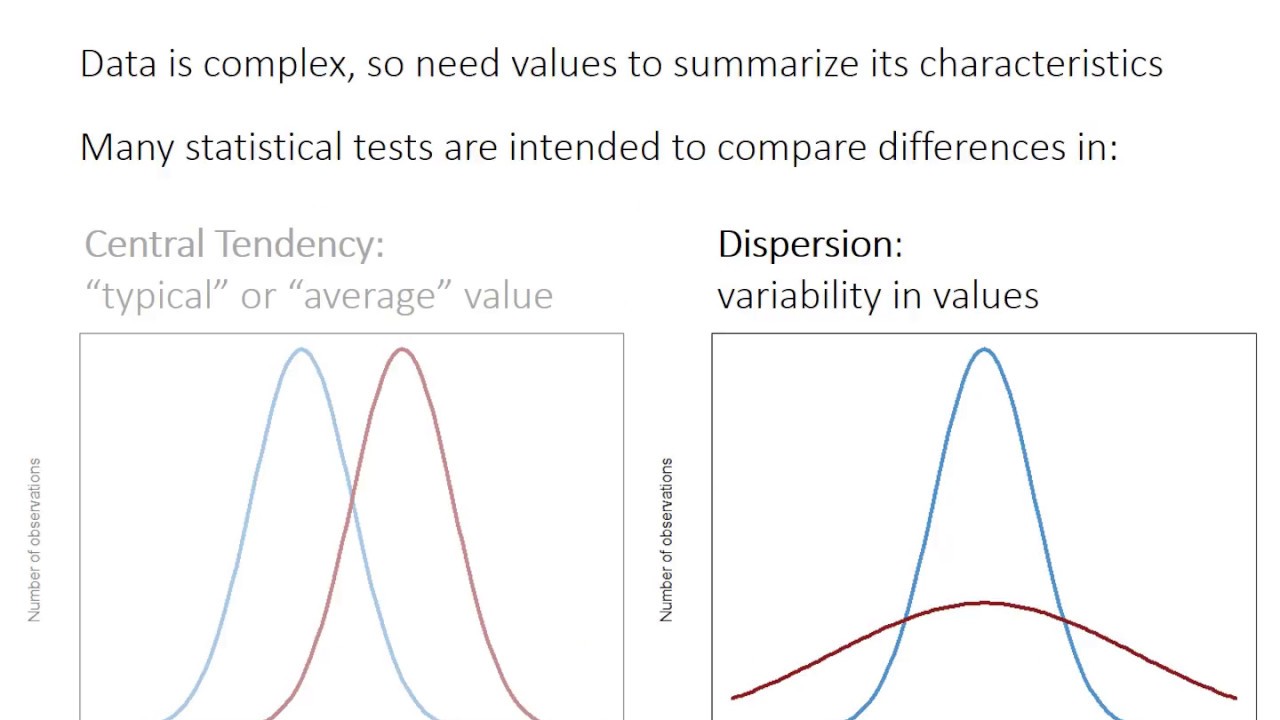
In high-throughput assays, this limitation can be overcome by pooling information across genes, specifically, by exploiting assumptions about the similarity of the variances of different genes measured in the same experiment. Inferential methods that treat each gene separately suffer here from lack of power, due to the high uncertainty of within-group variance estimates. This task is general: methods for it are typically also applicable for other comparative HTS assays, including chromatin immunoprecipitation sequencing, chromosome conformation capture, or counting observed taxa in metagenomic studies.īesides the need to account for the specifics of count data, such as non-normality and a dependence of the variance on the mean, a core challenge is the small number of samples in typical HTS experiments – often as few as two or three replicates per condition. An important task here is the analysis of RNA sequencing (RNA-seq) data with the aim of finding genes that are differentially expressed across groups of samples. The rapid adoption of high-throughput sequencing (HTS) technologies for genomic studies has resulted in a need for statistical methods to assess quantitative differences between experiments.
